Enhancing Road Safety Through Data Annotation
Project Type
Project Length
May, 2020 - Mar, 2024
Industry
IT Services
The annotations provided were incredibly detailed and accurate, offering us a high-quality dataset that was crucial for training our machine learning models.
Spokesperson, Software Company
Key Results
1000+ images annotated
15000+ annotations added
97% accuracy rate achieved
Project Overview
The project was designed to annotate a wide variety of categories, including surfaces, damages, markings, and signs, using a tool akin to Dataturks. The primary objective was to create a comprehensive and accurately labeled dataset that would serve as a foundation for a machine learning-driven product. This product was intended to identify and assess damages, interpret signs, and evaluate conditions across various environments. By meticulously categorizing and labeling these diverse elements, the project aimed to enhance the capability of the machine learning model to analyze and recognize different types of data effectively, thereby improving its performance and accuracy in real-world applications.

Business Problem
The project addressed a critical challenge in the field of machine learning by focusing on the accurate annotation of images to support the development of algorithms for detecting damages and analyzing surface conditions. This involved a detailed and precise process of identifying and outlining various features within the images, including roads, markings, and signs. The accuracy of these annotations was essential to ensuring that the machine learning models trained on this data could effectively recognize and interpret road conditions. By providing meticulously labeled images, the project aimed to enhance the quality and reliability of the machine learning models, ultimately contributing to more accurate road damage detection and surface analysis.
Solutions Delivered to the Client
By delivering meticulously precise annotations, we provided the client with a high-quality, well-structured labeled dataset essential for training highly accurate machine learning models focused on damage detection and surface analysis. This dataset, featuring detailed and accurate annotations of various road features, including damages, markings, and signs, was crucial for enhancing the performance of the machine learning algorithms. The accuracy and comprehensiveness of the annotations ensured that the models could effectively learn and generalize from the data, leading to more reliable and precise detection of road conditions. This contribution was key in supporting the client's efforts to develop advanced technologies for monitoring and maintaining road infrastructure, ultimately leading to improved safety and operational efficiency.

People involved
Mariia
Project Manager

Yuliya
Internet Research Expert
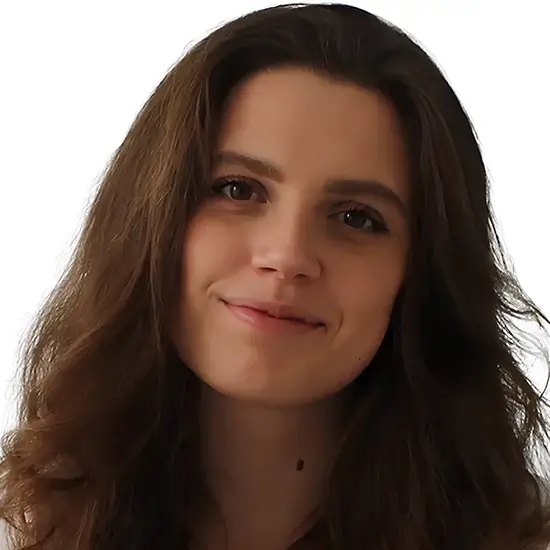
Iryna
Internet Research Expert
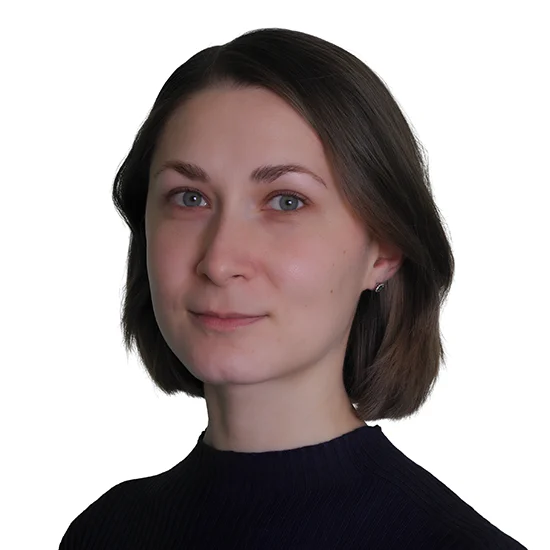